Research
overview | systems | climate | land use | land cover
We
are using different approaches and quantitative tools to generate
a set of plausible land use/cover change scenarios for East Africa.
Results of our analysis and synthesis will be an assessment, through
the historical interpretation of land use change, of a range of
plausible future scenarios which can be linked to land surface
properties required by the regional climate model.
Expert
Consensus of Future Land Cover Scenarios for East Africa.
In order to allow the climate modelers to begin work early in
the project, the land use team will use a current land cover map,
population change data and maps of the biophysical attributes
(e.g., elevation, agroecological zones) to develop two future
(year 2020) land cover digital maps using the GIS and expert judgment.
We will attempt to determine where agriculture will expand, urban
areas expand, where forests will be lost and where wildlife preserves
threatened.
Analysis
of Drivers of Land Use Change from Case Studies.
The land use/ cover change analysis of driving forces will be informed
by past research on the historical context, including political,
economic, social and biophysical factors, within which land use
change has occurred. This context has been derived from long-term
socioeconomic and biophysical field research in our case study
sites across the East Africa region. The research methods include
household, agricultural, soil and vegetative surveys in transects
crossing altitudinal gradients, interpretation of aerial photographs
and satellite imagery, and consulting secondary sources such as
population censuses, agricultural statistics and other literature.
The research has focused on social/ environmental interaction
issues such as the impact of land use change on ecosystems and
soil degradation, and the impact of drought on pastoral communities.
Model
Land Use Scenarios for the East Africa Region using Spatial Databases.
MSU’s Land
Transformation Model (LTM) is a spatial allocation tool that
can be used to assess variables associated with of historical
land use change (Pijanowski et al., 2001a; 2001b; 2002). The models
uses neural nets which train on data processed by a GIS to numerically
solve spatial interactions between surrogates (e.g., distance
from the nearest road, size of a parcel) of land use change drivers.
The model has several strengths. First, very few spatial drivers
(e.g., 3-7) can be used to build an accurate model. Second, neural
nets are able to generalize across datasets and across spatial
regions (Pijanowski et al., 2001) and thus can be a useful tool
to scaling up from small training sets. Third, it has been already
shown (Pijanowski, Olson, and Campbell, in prep.) to perform very
well on predicting locations of historical agricultural extensification
in two key East African case study areas: Embu/Mbeere and Loitokitok.
We
will use the LTM to explore how the information from the case
study areas can be used to create forecasts of land use across
East Africa. Information on time series land use change from case
study sites will be used to conduct “learning exercises”
following Pijanowski et al. (1991a) that will establish a set
of initial parameters (e.g., distance from nearest town, elevation)
for the model. Once the initial LTM is established, the team will
introduce information from time series climate data to simulate
the impact of climate change on land use. The introduction of
climatic data represents an innovation in such modeling.
|
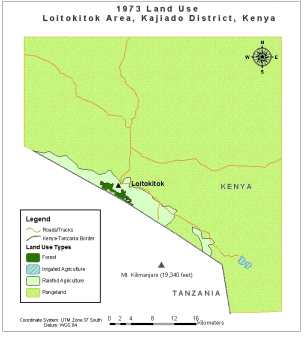
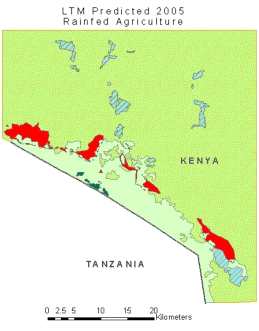
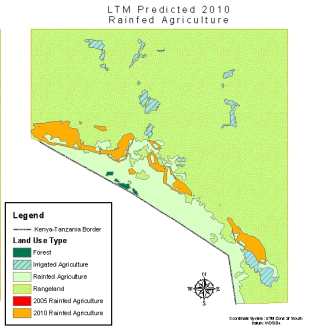
|